Building Tomorrow's AI Infrastructure: The Data Center Revolution
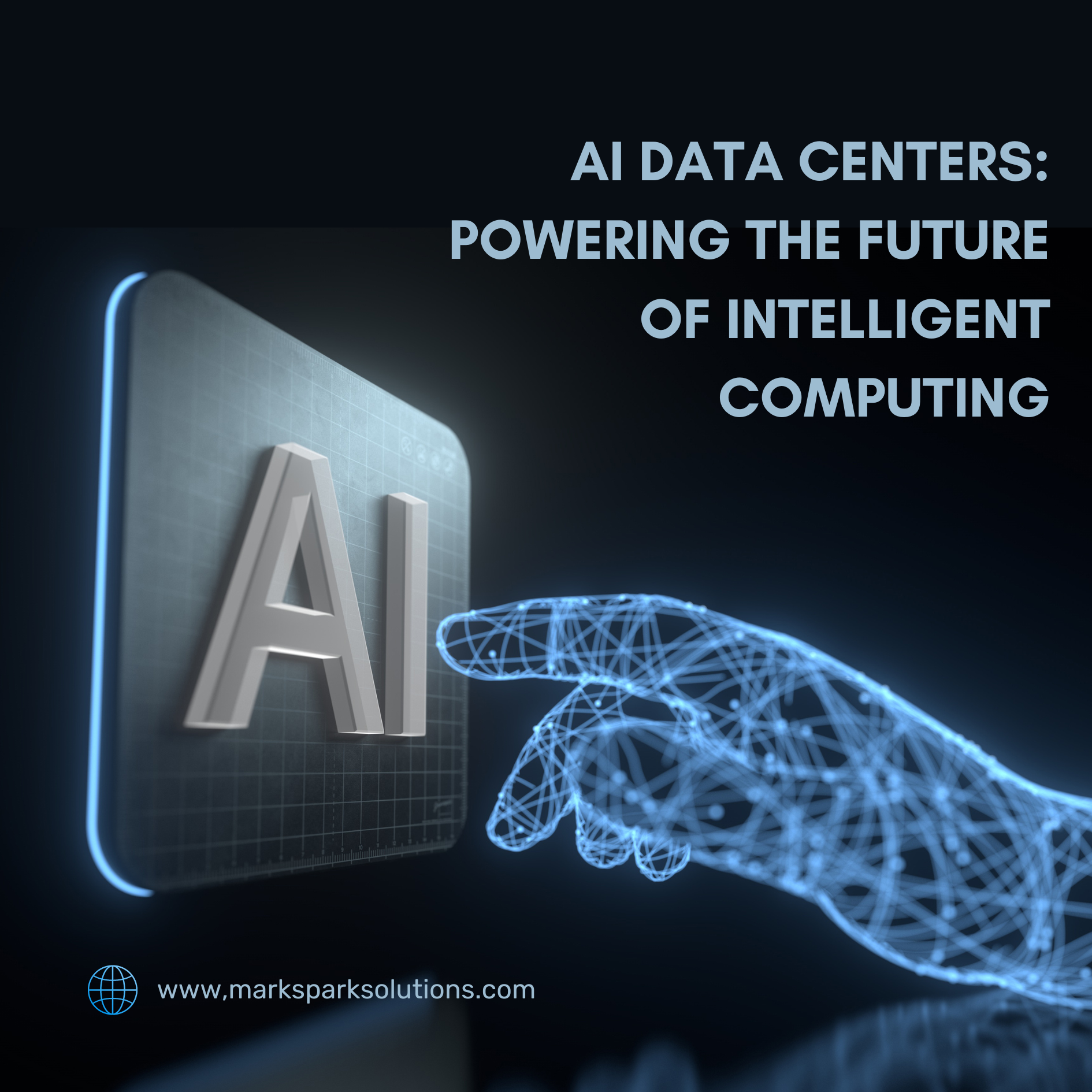
As artificial intelligence (AI) becomes more integrated into modern industries, the demand for data centers specifically designed to handle the processing, storage, and management of AI workloads is increasing. The AI data center market is growing rapidly due to the need for advanced computing power, real-time data processing, and improved infrastructure to support AI applications. AI workloads, such as machine learning (ML), deep learning, and natural language processing (NLP), require significant computational resources, often surpassing the capacity of traditional data centers. As AI applications expand, the demand for specialized data centers is also growing, leading to increased investment in innovative technologies and infrastructure.
Key Technologies Powering AI Data Centers
To support AI operations, data centers need to adopt advanced technologies that provide scalable, high-performance computing environments. Some of the key potential technologies transforming the AI data center landscape include:
Graphics Processing Units (GPUs): Essential for parallel processing tasks, GPUs are critical for training and deploying AI models. NVIDIA, AMD, and Intel dominate the GPU market for AI applications.
Tensor Processing Units (TPUs): Developed by Google, TPUs are optimized for machine learning workloads, particularly in executing neural network algorithms. They are widely used in data centers catering to AI applications.
Quantum Computing: Quantum computing, though still in its early stages, holds immense potential for AI by significantly speeding up complex computations. Companies like IBM and D-Wave are leading in quantum computing research.
Optical Interconnects: With the rise of high-performance computing, optical communication within data centers is becoming critical. Photonic technologies can enhance data transmission speeds, which is vital for AI data centers processing massive volumes of data.
AI-Driven Data Center Automation: AI is being used to optimize data center operations, reducing energy consumption, optimizing cooling systems, and improving the overall efficiency of resource utilization.
Investment in AI Data Centers
Global investments in AI data centers are increasing rapidly, driven by the exponential growth of AI-driven applications across industries such as healthcare, automotive, finance, and retail. These investments cover hardware, software, and infrastructure development. Below is a table highlighting some of the key investments made in AI data center technologies:
Technology |
Investment (in USD billions) |
Investment Area |
GPUs |
10 |
Hardware for AI model training and inference |
TPUs |
5 |
Specialized chips for neural network computations |
Quantum Computing |
2 |
Research and development in AI processing capabilities |
AI-Driven Data Center Automation |
3 |
Operational efficiency and energy optimization |
Optical Interconnects |
1.5 |
High-speed data transmission for large-scale AI workloads |
Major Companies Operating in the AI Data Center Market
Several global giants dominate the AI data center space, with both traditional data center operators and AI-centric companies investing heavily in this growing market. These companies are involved in developing AI-specific hardware, building and expanding data center infrastructure, and offering cloud-based AI solutions. Here’s a breakdown of the key players and their recent investments:
Company |
Recent AI Data Center Investment (in USD billions) |
Focus Area |
Google Cloud |
4.5 |
AI-specific TPUs, cloud AI services |
Amazon Web Services (AWS) |
6 |
AI cloud services, GPU/TPU infrastructure |
Microsoft Azure |
5.8 |
AI and ML cloud platform, AI-driven automation |
NVIDIA |
10 |
GPUs for AI model training and deployment |
IBM |
3 |
Quantum computing for AI and cloud AI services |
The AI data center market is growing rapidly, but it faces several challenges that could slow its development and widespread adoption:
- Energy Consumption: AI workloads, especially deep learning tasks, require a lot of energy. This could have a significant environmental impact, as data centers already consume about 1% of the world's electricity. Energy-efficient solutions are needed for sustainable growth.
- Data Management and Security: AI data centers handle massive amounts of data, including sensitive information. Protecting the privacy and security of this data is a major concern due to strict regulations like GDPR. Data breaches could be catastrophic.
- High Infrastructure Costs: Building AI data centers requires significant capital investment for advanced hardware, cooling systems, and power supplies. The rapid evolution of AI technologies means ongoing investment is needed to stay competitive.
- Latency Issues: Many AI applications need ultra-low latency for real-time decision-making. Traditional data centers struggle with this, but edge computing solutions, which process data closer to the source, are emerging as a solution.
- Talent Shortage: There is a global shortage of skilled AI professionals and data center engineers. The complexity of designing and managing AI-specific data centers requires highly specialized knowledge.
In conclusion, while the AI data center market has great potential, addressing these challenges will require innovative solutions such as AI-driven automation, edge computing, and quantum computing. Overcoming these hurdles will be crucial for the future of computing.